Designing hybrid human-AI use cases for the enterprise
How should we approach designing and implementing hybrid use cases that involve GenAI + new ways of working + human oversight in the enterprise ?
GPTs: fun, but also useful?
Azeem Azhar is doing a great job of documenting and analysing the rapid development of GenAI in his excellent newsletter Exponential View, and last week he shared a discussion with one of the co-authors of the original Transformers paper (Attention is all you need), Aidan Gomez, whose company Cohere is trying to advance enterprise AI deployment.
It was a very informative and thoughtful chat, but what struck me most of all was how hard it was to articulate concrete enterprise AI use cases that might drive adoption, as opposed to proofs of concept and sandbox deployments that could lead to use case discovery. The pull-quote Azeem used at the start of the video reflected this:
“It's very rare that in isolation an LLM is actually useful - very rare; it can be fun […] to just chat to a model and it can be pretty good at helping you write stuff so […] it could help you do copywriting, but overwhelmingly, I think the real value we'll find from LLMs is in plugging them into broader systems”
The current pace of GPT development is breathtaking, with talk of GPT-5 being a leap forward comparable to GPT-4, new approaches to using Retrieval Augmented Generation (RAG) and Retrieval-augmented Fine Tuning (RAFT) to improve results based on local data, and also (as Aidan mentioned) a big focus on scaling the models.
There will be a lot more that AI pioneers can do to clean data, improve weightings, fine-tune algorithms and improve curation to improve GenAI results, but LLMs are fundamentally a knowledge architecture, not a reasoning or planning architecture, and perhaps we need more focus on autonomous agents and embedding LLM capabilities inside higher-order business capabilities or ‘apps’.
Better at hard tasks than easy (human) ones
Right now, GPTs are great at wowing people with their ability to synthesise knowledge about anything you can imagine, and also useful in very constrained language domains such as medicine, professional services and coding. AI pioneer Andrej Karpathy tweeted recently that he believes autonomous coding will soon emerge in a similar way to autonomous driving - i.e. starting with co-piloting and then gradually reducing human oversight as the system becomes more reliable. In fact, it might be that coding based on prompts is an easier problem to solve than autonomous driving.
But routine use of AI in the workplace, especially in an enterprise context, lies somewhere in the middle. It needs to be both a general synthesiser of knowledge, but also very specific and precise in certain domains and contexts where hallucinating could be dangerous.
The WSJ recently shared some interesting results from a large consulting firm’s experiment using GPTs with consultantsthat suggest it is not yet easy to predict where GPTs will work best, based on role or skill level:
On the creative product innovation task, humans supported by AI were much better than humans. But for the problem solving task, [the combination of] human and AI was not even equal to humans … meaning that AI was able to persuade humans, who are well known for their critical thinking … of something that was wrong.
AI excels at some individual tasks that humans find hard, but is not yet able to perform a wide variety of tasks that even an infant (or a dog) find so easy they do not need to think about them, and especially tasks that chain together multiple actions.
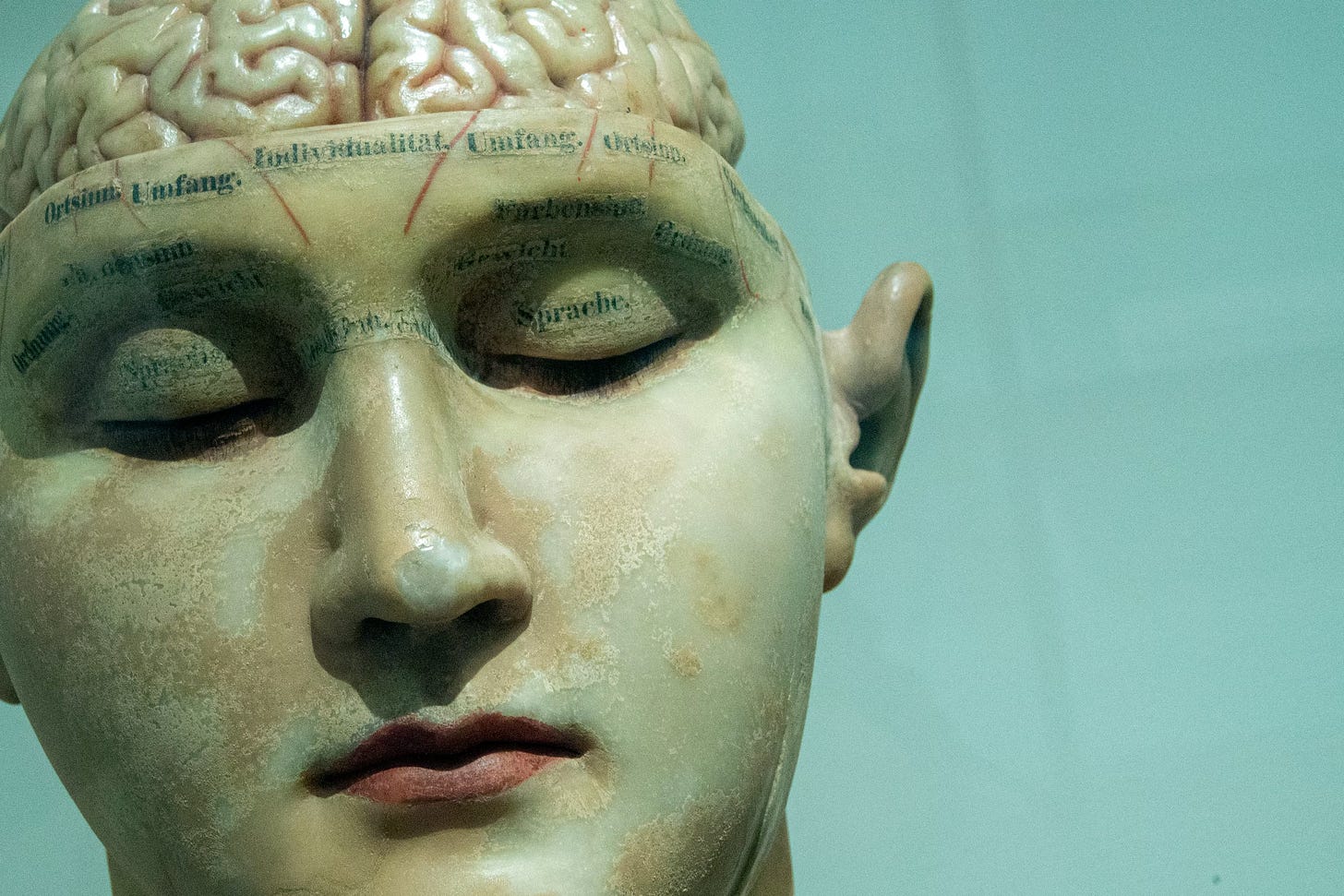
Designing hybrid human-AI use cases
Some critics of the current generation of AI and GPTs believe we will reach the limits of the ‘stochastic parrot’ approach and face another AI winter, as in the late C20th. Others, such as Filip Piekniewski argue for a more bottom-up approach informed by biological evolution, and believe ‘true’ intelligence is embodied and distributed, in addition to the neocortical thinking that GPTs are trying to replicate:
AI uses expressions like "data", "classification", "distribution", "association", "segmentation", "gradient optimization" ... AI rarely if ever uses expressions like "control loop", "feedback across scales", "scale-free composing", "self similarity", "control across time/space scales". You just won't find these words in any modern AI papers (you will find them in some robotics papers, but not strictly AI). It is a discipline which has stolen a sexy name, but expresses little interest with understanding how true intelligence arises or even how to define it
Piekniewski believes we need a different path, one that starts at the level of the ‘atom of intelligence’ and gradually evolves by abstracting and scaling in a more ‘natural’ way until it reaches the level of the human autonomic nervous system and its relationship with the brain(s).
But a more attainable near-term goal might be to focus more on human-supervised learning and composing GPTs, LLMs, simple agents and automations into higher order business capabilities that teams own and manage - i.e. with a co-pilot at the wheel providing insight and oversight.
For each AI ‘skill’ or app, we need to integrate with other work, knowledge, data and human input - bundles of integrated human and machine activities wrapped in process or workflows.
There are, of course, some simple, single uses of LLMs, such as summarising research, helping create content objects or answering questions, and this is where a lot of early focus will be applied with co-pilots and RAG GPTs that have access to corporate knowledge and data stores, directories and so on.
But as with so many other apparently transformative digital technologies, implementing them within existing business structures offers incremental gains at best. The real prize is making possible substantially smarter, cheaper and more connected operating models by combining technologies such as GPTs with new digital ways of working and managing.
A new approach to management and learning?
We need a complete re-write of management doctrine and a re-invention of learning if we are to make the most of AI in the enterprise.
Proponents of modern, agile leadership have argued for years that leaders need to be navigators and system thinkers, not KPI-led micro-managers, if our organisations are to enjoy greater autonomy, agility and innovative potential. But in a world of humans working with AI at the task level, plus foundational machine learning and smart data at the platform level, this transition becomes even more salient.
The implications for learning are just as great. Prompting, reviewing and guiding AIs demands a whole new type of literacy. Just as programming is about imagining a goal and articulating the instructions to persuade a computer to achieve it, prompting will become a powerful language of guiding and controlling systems and processes. But it also demands critical thinking and planning skills to both evaluate the output of AIs and discern truth from hallucination.
Right now we are in the uncanny valley, discovering ways to get some sense out of these moody apparitions, as Ethan Mollick wrote about a couple of weeks ago:
What is the most effective way to prompt Meta’s open source Llama 2 AI to do math accurately? Take a moment to try to guess.
Whatever you guessed, I can say with confidence that you are wrong. The right answer is to pretend to be in a Star Trek episode or a political thriller, depending on how many math questions you want the AI to answer.
McKinsey just released an interesting study of employer and employee perceptions of AI, and asked whether AI could make companies more people-centric:
As teams start using gen AI to help free up their capacity, the middle manager’s job will evolve to managing both people and the use of this technology to enhance their output. In other words, gen AI will become another member of the team to be managed. And just like a direct report who needs some intensive coaching to get up to speed, gen AI may need more guidance and involvement from managers—at least initially and perhaps for much longer.
The level of change needed is exhilarating, and I think we will see human-AI smart organisations outperform traditional bureaucratic organisations before long.
Announcement: how we plan to help
It is not enough to describe an AI-driven use case for the enterprise without reference to the new ways of working, process changes, capabilities and skills needed to make it work.
We have been working on this challenge for some time, developing interventions that are more actionable than executive learning, more engaging than change management, and more strategically valuable than external consulting and advisory.
We will shortly be launching our new venture, Shift*Base, whose mission is to be a digital capability accelerator for organisations, and ultimately a development environment (like an IDE for new organisational operating systems. The platform can be used by leaders with their teams to map, connect and improve emerging digital business capabilities, launch change missions around them, and support that process with customised learning, guides and implementation playbooks.
Also, in recognition of the sheer impossibility of keeping up with all aspects of this fast moving field that sits at the nexus of AI development, organisational change and new ways of working, we will refocus this newsletter to become a personal learning companion for leaders at every level, from teams to C-suites, with more practical implementation content such as capability maps, recipes, playbooks and guides, in addition to the newsletter elements that try to make sense of current developments.
In recognition of your interest and support, we will give existing LinkLog subscribers free access to what will become a paid premium version for 6 issues (new joiners will get 3 issues), as well as the ability to unlock further free access by referring executives and practitioners who you think could benefit from this content.
The first re-launched edition will be sent around April 2, and will likely come from a new address: academy@shiftbase.info so please add this to your white list if you can and please help spread the word!