Strawberry (Da)Queries, Machines and Possibilitarianism
Analysis of OpenAI's first o1 series release and why this does not risk de-humanising the enterprise - quite the opposite, in fact...
Strawberry (da)queries are more sophisticated than single shot prompts
The big news in GenAI this weekend was the launch of GPT o1-preview, aka Strawberry, which is capable of limited reasoning and thinking through problems from different angles before trying to solve them. Whilst its underlying LLM capabilities are not much different from GPT 4.0, the additional thinking loop makes Strawberry more suitable for multi-stage or complex questions that need planning and reflection on possible solutions.
Nathan Lambert’s deep dive into o1 is worth a read if you really want to understand the technical detail behind this new model, but a few things stand out:
This is a sophisticated model optimised for complex tasks that require Chain of Thought (CoT) reasoning and self-play reinforcement learning (similar to that used to beat games such as Go) and takes longer and uses many more tokens to achieve a result, so it is not for every task.
It does a lot more of the clever stuff in real-time, such as inference and testing, whereas previous models did most of their work in pre- and post-training before release.
It can self-correct to a certain extent by scoring multiple future reasoning steps using a reward model to avoid making obvious mistakes, which seems to be a step forward in avoiding some forms of hallucination.
OpenAI’s reported benchmarks for the model certainly show a significant improvement on GPT4o:
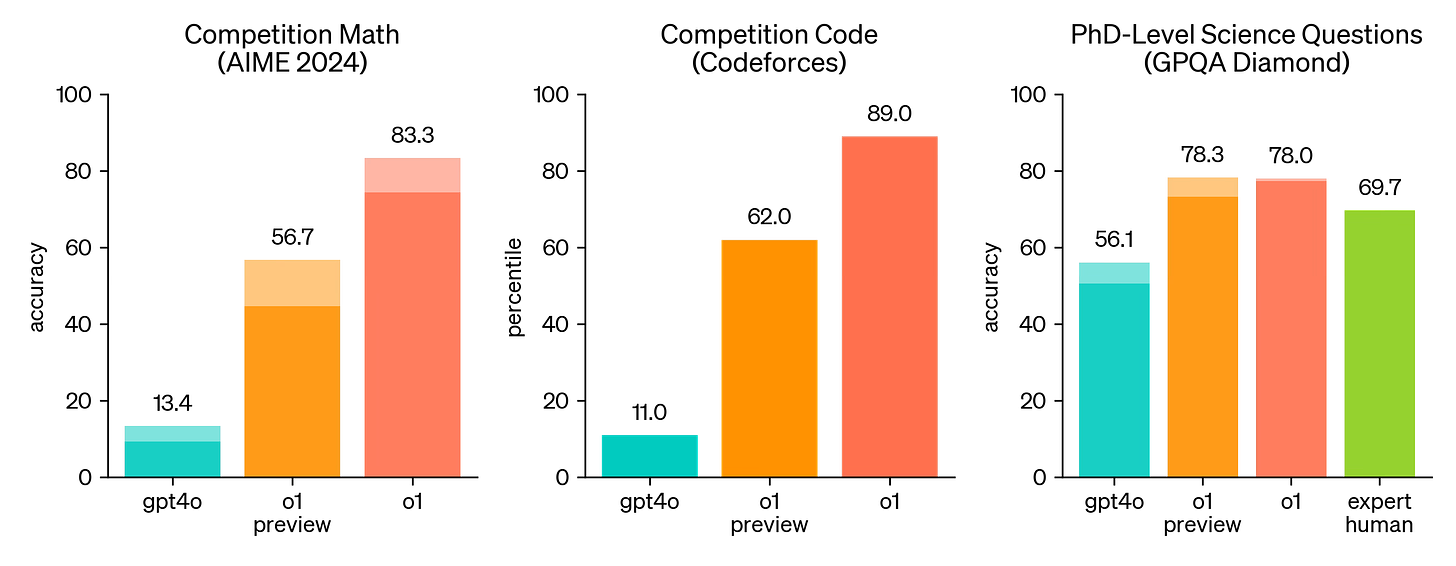
Ethan Mollick, who has had preview access for some time, was impressed, concluding:
Using o1-preview means confronting a paradigm change in AI. Planning is a form of agency, where the AI arrives at conclusions about how to solve a problem on its own, without our help. You can see from the video above that the AI does so much thinking and heavy lifting, churning out complete results, that my role as a human partner feels diminished. It just does its thing and hands me an answer. Sure, I can sift through its pages of reasoning to spot mistakes, but I no longer feel as connected to the AI output, or that I am playing as large a role in shaping where the solution is going. This isn’t necessarily bad, but it is different.
Maxim Lott suggests this development has effectively increased the IQ-equivalence of an LLM by 30 points to 120, based on its ability to answer questions on the Norway Mensa IQ test.
Azeem Azhar also covered Strawberry in Exponential view and concluded that organisations will soon need to reset their expectations of what problems can be solved in future. He also made reference to studies of the different philosophical approaches underpinning Babylonian science (based on extrapolation) and Athenian science (more creative-speculative) as an example of how different starting assumptions about discovery and possibility can have a big impact on where we end up.
Judging AIs using IQ tests is probably as reductive and limited for machines as it has been for evaluating human intelligence, but the creative possibilities of people (or teams of people) using AIs that are capable of reasoning and dialogue is undeniably exciting. But we need to find the right balance between improving what we do today versus re-imagining how we do things to make a real step change in value creation and work.
Putting the body back into corporate
Will this de-humanise our organisations? Not necessarily.
At its worst, the bureaucratic corporation is like a machine made of meat, with neither the efficiency of a real machine, nor the insights and ingenuity of humans. Management orthodoxy has pursued artificial stupidity for so long, in the form of unthinking process management compliance and the suppression of experimentation and creativity, that it is no wonder AI can replace what are already robotic roles. We can do better.
Klarna recently trumpeted its AI-led headcount reductions in customer service roles, and now Salesforce is making a big bet on Agentforce - a system of AI agents that can pursue higher order goals beyond simple chatbots for sales and customer service (see also their very interesting research on the large action models that make this possible).
There is nothing wrong with these use cases, but in the bigger picture, AI presents opportunities for organisations to be more machine-like and automated in their infrastructure, precisely to create the space and freedom for people to think and work as humans using that infrastructure. That will be the game changer, not better call centres.
Medieval historian Cristian Ispir reminded us about the origins of the term ‘corporate’ this week:
In the medieval period, the idea of a corporation wasn't about shareholder value or quarterly profits; it was about flesh and blood, a community bound together as a single "body"—a corpus.
Guilds of bakers, masons, and merchants were not just economic entities but living, breathing collectives with a shared purpose, a common pulse, and a clear role in the social fabric.
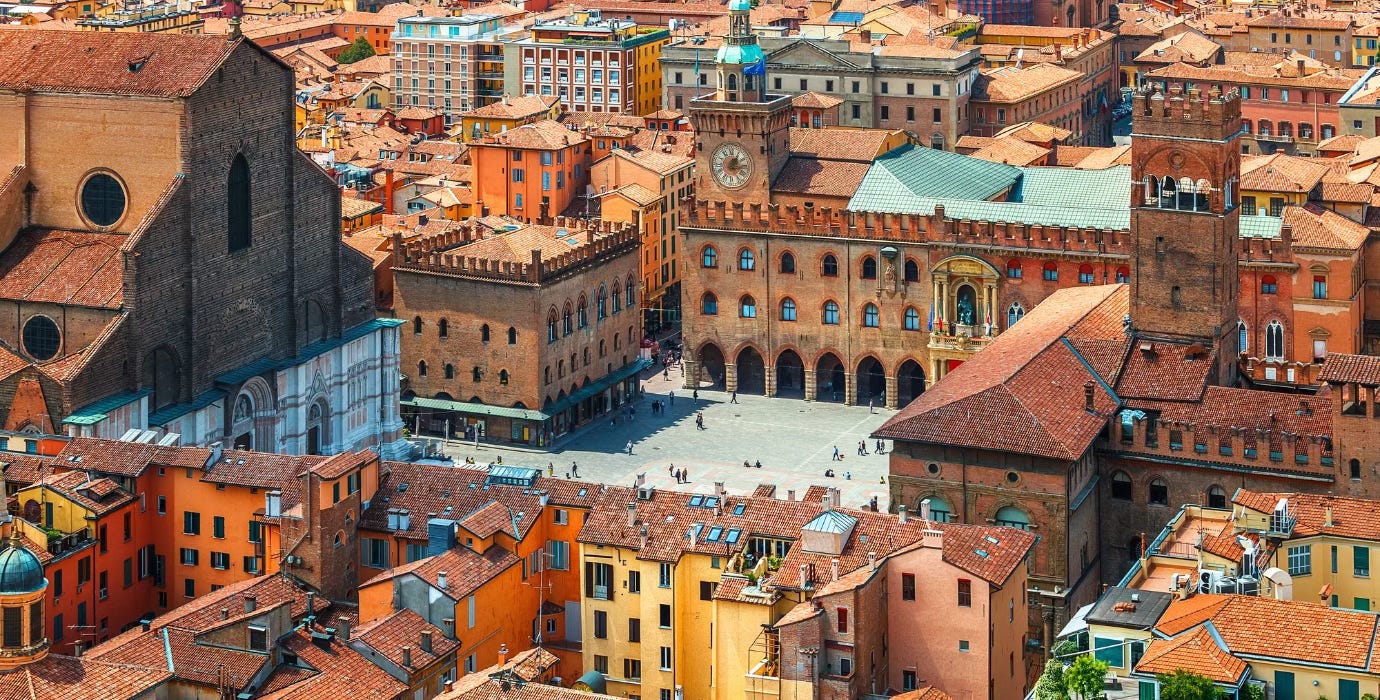
All organisations exist to serve people, whether their owners, workers, customers or wider society, and AI gives us the chance to re-balance that relationship in a positive way between the organisation’s administration and infrastructure on the one hand, and its people on the other. We can expect to see smaller teams achieving much more at a radically lower cost of management overhead, thanks to smart tech systems and platforms that act as force multipliers and simplify coordination.
Even at a basic level, OpenAI’s advances will soon filter through to improvements in the Microsoft digital workplace stack, with Co-Pilot Agents and Co-Pilot pages, which will bring AI augmentation to collaborative content in Loop and SharePoint.
David Heinemeier Hansson shared an interesting tongue-in-cheek provocation recently: Optimize for bio cores first, silicon cores second, arguing:
… the price of one biological core [assuming $200k pa for a US programmer] is the same as the price of 3663 silicon cores. Meaning that if you manage to make the bio core 10% more efficient, you will have saved the equivalent cost of 366 silicon cores. Make the bio core a quarter more efficient, and you'll have saved nearly ONE THOUSAND silicon cores!
So whenever you hear a discussion about computing efficiency, you should always have the squishy, biological cores in mind. Most software around the world is priced on their inputs, not on the silicon it requires. Meaning even small incremental improvements to bio core productivity is worth large additional expenditures on silicon chips. And every year, the ratio grows greater in favor of the bio cores.
See also his recent piece about how these biological computing cores at 37Signals no longer have any managers but rather distribute management tasks among all senior contributors to avoid this work expanding to fill entire roles. Given how many basic managerial coordination tasks are ripe for automation, I expect we will see more of this.
Possibilitarianism
So much of what will determine the most impactful uses of enterprise AI in the context of abundant synthetic intelligence will come down to asking better questions, as I wrote two weeks ago, which, like first principles thinking, is a higher order skill that the best leaders tend to possess.
For leaders trying to find where and how to try AI in the enterprise, a good direction of travel is to let machines do machine stuff, and use smart tech and collaborative working to elevate the human. After all, GenAI is essentially a social knowledge machine trained on our collective outpourings / wisdom (delete as appropriate), especially when using Retrieval Augmented Generation (RAG) based on an organisation’s own content, so in a sense we are learning from ourselves.
But we will need to unlearn a lot of learned helplessness in the enterprise, and broaden our sense of the possible if we are to really take advantage of AI and smart tech rather than just automating old ways of doing things.
We need more possibilitarians, which is what Dave Gray has been working on with his school of the possible. If we are to be more Athenian in our hypotheses and ready to explore new horizons rather than plan and extrapolate from yesterday’s reality, then we will get a lot more out of the coming era of AI agents than if we try to wrap the tech around existing management systems and methods.
If you would like to learn more about our introductory workshops and immersions on practical enterprise AI use cases, please contact us for more information